Jun 27, 2024
Implementing AI Strategy in U.S. Businesses
SUBSCRIBE
Introduction
The rapid advancements in Artificial Intelligence (AI) have heralded a new era of innovation, fundamentally transforming the landscape of modern business. From enhancing operational efficiency to enabling data-driven decision-making, AI presents an unprecedented opportunity for businesses to gain a competitive edge. In the U.S., companies across various sectors are increasingly recognizing the transformative potential of AI and are keen on integrating it into their strategic frameworks.
This comprehensive guide explores how businesses can effectively implement an AI strategy, ensuring alignment with organizational goals, robust infrastructure, skilled talent, and ethical governance. By examining strategic planning, infrastructure requirements, data strategy, talent development, project implementation, and the measurement of AI impact, we aim to provide a roadmap for U.S. businesses to navigate the complexities of AI adoption and maximize its benefits.
Strategic Planning for AI

Defining the Vision and Scope
The first step in implementing an AI strategy is to define a clear vision and scope for AI initiatives. This involves identifying the specific business problems that AI can solve and the goals it aims to achieve. The vision should be aligned with the overall business strategy, ensuring that AI projects support the broader objectives of the organization.
A well-defined vision provides direction and helps in prioritizing AI use cases. For instance, a retail company might focus on using AI for personalized customer experiences, while a manufacturing firm might prioritize predictive maintenance to reduce downtime. The vision should be specific enough to provide a clear roadmap but flexible enough to accommodate new opportunities as they arise.
Steps to Define Vision and Scope:
Identify Key Business Challenges: Start by pinpointing the critical challenges and opportunities within your business that AI could address. This could include improving customer engagement, enhancing supply chain efficiency, or predicting market trends.
Set Clear Objectives: Define what success looks like for your AI initiatives.
Engage Stakeholders: Involve key stakeholders from various departments to ensure that the vision and scope are comprehensive and aligned with the company's strategic goals.
Document the Vision: Create a detailed document that outlines the AI vision, including the rationale, expected benefits, and high-level implementation plan.
Aligning AI Initiatives with Business Goals

Alignment with business goals is crucial for the success of AI projects. This requires collaboration between AI experts and business leaders to ensure that AI initiatives are integrated into the strategic planning process. Business leaders should understand the potential of AI and how it can drive value in their specific context.
Steps to Align AI Initiatives:
Cross-Functional Collaboration: Establish cross-functional teams that include members from IT, operations, marketing, finance, and other relevant departments. This promotes diverse perspectives and ensures that AI initiatives are relevant to different parts of the business.
Executive Sponsorship: Secure commitment and sponsorship from senior leadership to ensure that AI projects receive the necessary resources and support.
Continuous Communication: Maintain ongoing communication between AI teams and business units to ensure alignment and address any emerging challenges promptly.
Performance Metrics: Develop metrics to measure the impact of AI initiatives on business goals. Regularly review these metrics to ensure that AI projects are delivering the expected value.
Prioritizing AI Use Cases and Projects
Not all AI projects are created equal, and businesses must prioritize the ones that offer the highest return on investment (ROI). Prioritization should be based on factors such as potential business impact, feasibility, cost, and time to implement. A phased approach, starting with pilot projects, can help in testing the viability of AI solutions before scaling them across the organization.
Steps to Prioritize AI Projects:
Evaluate Business Impact: Assess the potential impact of each AI use case on business performance. Focus on projects that can significantly enhance revenue, reduce costs, or improve customer satisfaction.
Assess Feasibility: Consider the technical feasibility of implementing each AI project. Evaluate factors such as data availability, technical complexity, and resource requirements.
Calculate ROI: Estimate the costs and benefits of each AI project to determine the expected ROI. Prioritize projects with the highest ROI and shortest payback period.
Pilot and Scale: Start with pilot projects to test AI solutions on a small scale. Use the learnings from pilot projects to refine the approach and plan for larger-scale deployments.
Example: A financial services company might start with an AI pilot project for fraud detection. Once the pilot demonstrates success, the company can expand AI use to other areas such as customer service automation and risk management.
By following these strategic planning steps, businesses can ensure that their AI initiatives are well-defined, aligned with their goals, and prioritized for maximum impact.
Infrastructure and Technology

Building the Necessary IT Infrastructure
Implementing AI requires a robust IT infrastructure that can support the computational needs of AI models. This includes high-performance computing resources, data storage solutions, and networking capabilities. Cloud computing has emerged as a popular option for AI infrastructure due to its scalability and flexibility.
Businesses should evaluate their current IT infrastructure and identify gaps that need to be addressed. This might involve upgrading hardware, investing in cloud services, or enhancing cybersecurity measures to protect sensitive data.
Selecting AI Technologies and Platforms
Choosing the right AI technologies and platforms is critical for the success of AI projects. Businesses should consider factors such as compatibility with existing systems, scalability, ease of use, and vendor support. Open-source AI frameworks like TensorFlow and PyTorch offer flexibility and a wide range of pre-built models, while commercial AI platforms from companies like IBM, Google, and Microsoft provide comprehensive tools and services.
The selection process should involve thorough research, pilot testing, and consultations with AI experts to ensure that the chosen technologies meet the specific needs of the organization.
Integrating AI with Existing Business Systems
Integration with existing business systems is essential for the seamless deployment of AI solutions. This involves connecting AI models to data sources, enterprise applications, and workflows. Application Programming Interfaces (APIs) and middleware solutions can facilitate integration, enabling AI to interact with different systems and processes.
For instance, integrating an AI-powered customer service chatbot with a company's CRM system can provide real-time customer data, enhancing the chatbot's ability to resolve queries efficiently.
Data Strategy
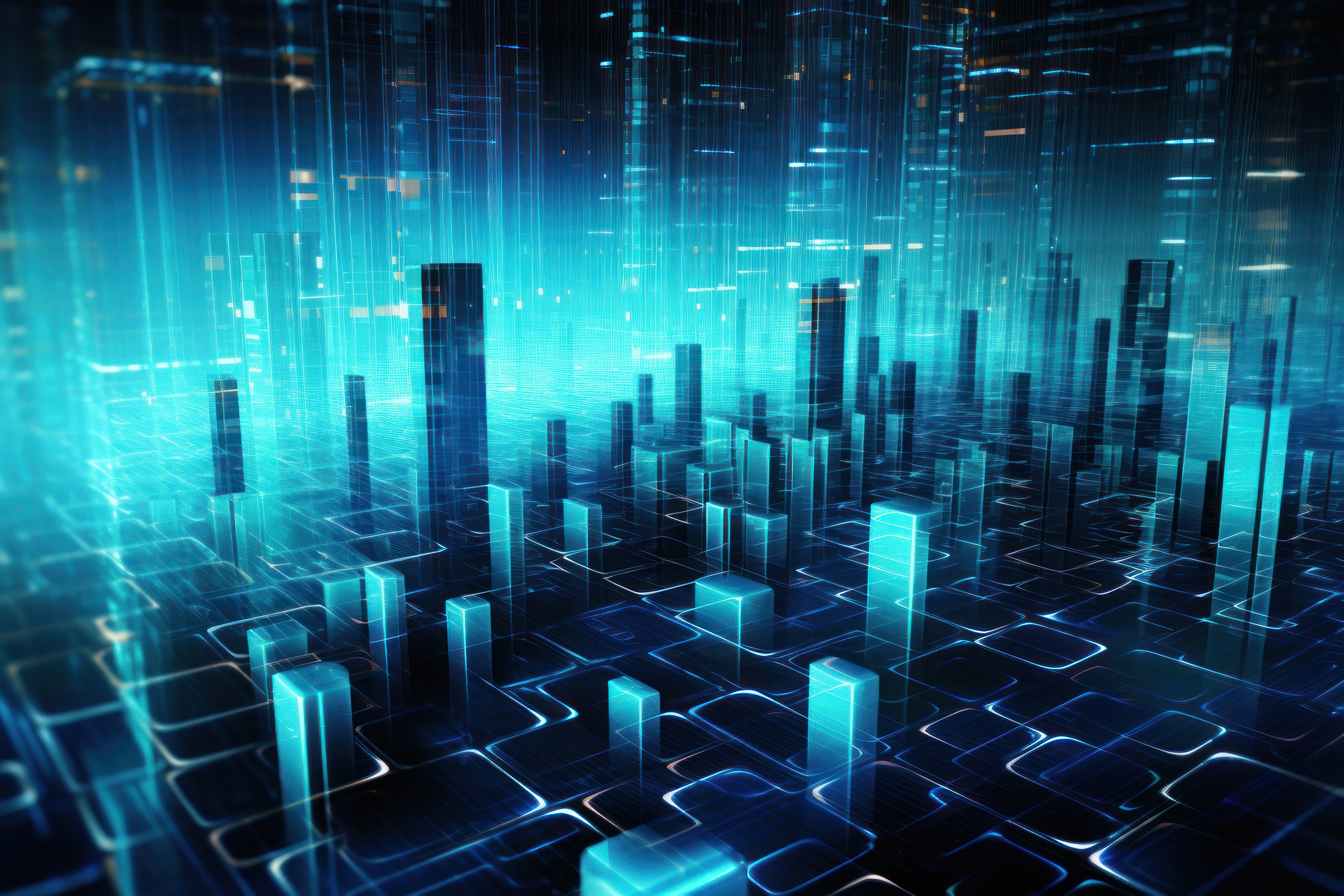
Developing a Robust Data Strategy
Data is the lifeblood of AI, and a robust data strategy is essential for successful AI implementation. This involves identifying the data required for AI projects, ensuring data quality, and establishing data governance frameworks. Businesses should define clear data policies and standards to maintain data integrity and compliance.
A comprehensive data strategy should also include data collection, storage, and management processes. This ensures that data is readily available for AI models and can be accessed securely and efficiently.
Ensuring Data Quality and Integrity
High-quality data is crucial for the accuracy and reliability of AI models. Businesses should invest in data cleaning and preprocessing techniques to remove errors, inconsistencies, and biases from their datasets. Data validation and verification processes should be implemented to ensure that data meets the required quality standards.
Regular audits and monitoring can help in maintaining data quality and identifying any issues that need to be addressed. This is particularly important for organizations that deal with large volumes of data from multiple sources.
Implementing Data Privacy and Security Measures
Data privacy and security are paramount when dealing with sensitive information. Businesses must comply with data protection regulations such as GDPR and CCPA and implement robust security measures to protect data from breaches and unauthorized access.
This involves encrypting data at rest and in transit, implementing access controls, and regularly updating security protocols. Employee training on data security best practices can also help in preventing data breaches and ensuring compliance with regulations.
Talent and Skills Development
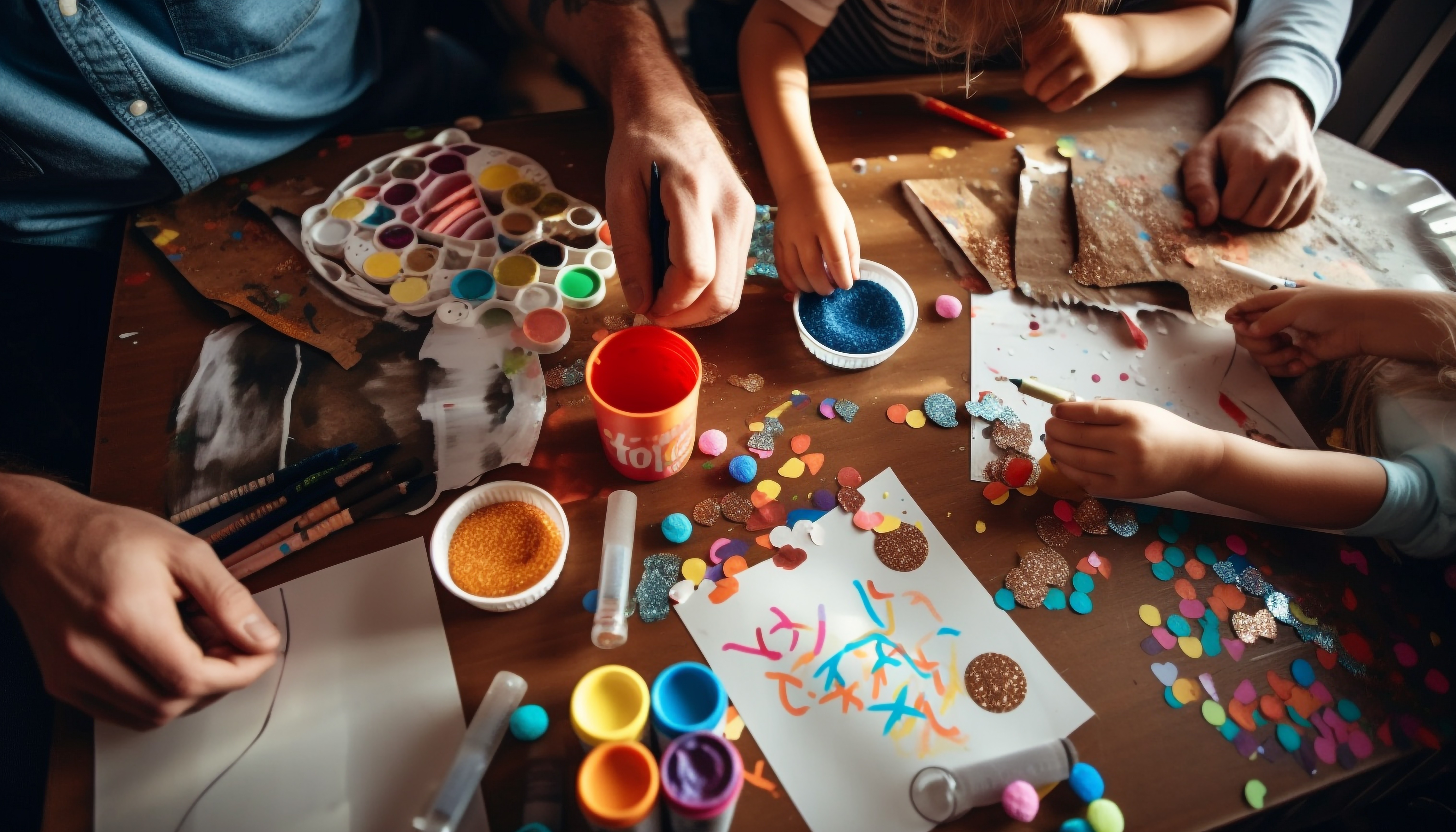
Identifying the Skills Needed for AI
AI implementation requires a diverse set of skills, including data science, machine learning, software development, and domain-specific expertise. Businesses should identify the skills needed for their AI projects and assess their current capabilities to identify gaps.
Hiring AI talent can be challenging due to the high demand for skilled professionals. Businesses should consider building internal capabilities by training existing employees and fostering a culture of continuous learning.
Training and Upskilling the Workforce
Training and upskilling the workforce are essential for the successful adoption of AI. This involves providing employees with the necessary knowledge and skills to work with AI technologies and understand their implications. Training programs can include workshops, online courses, and hands-on projects.
Collaboration with educational institutions and AI training providers can help businesses access the latest knowledge and best practices in AI. This can also provide opportunities for employees to earn certifications and credentials in AI-related fields.
Building a Culture of Continuous Learning and Innovation
A culture of continuous learning and innovation is crucial for staying competitive in the rapidly evolving field of AI. Businesses should encourage employees to experiment with new ideas, collaborate across departments, and share their knowledge and experiences.
Innovation labs and hackathons can provide a platform for employees to explore AI technologies and develop innovative solutions. Recognizing and rewarding innovation can further motivate employees to contribute to the organization's AI initiatives.
AI Project Implementation
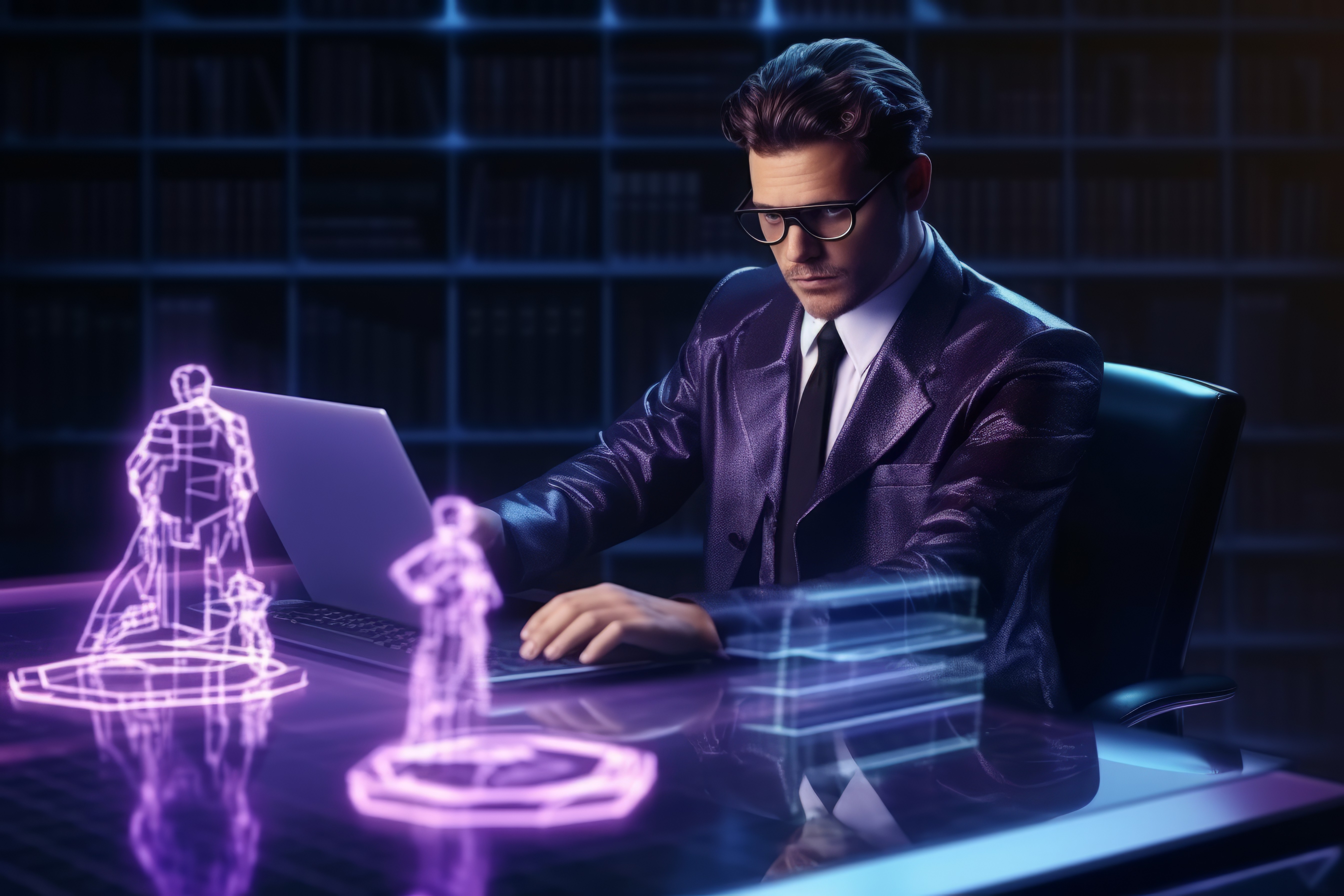
Designing and Deploying AI Models
Designing and deploying AI models involves selecting the appropriate algorithms, training the models on relevant datasets, and validating their performance. This requires a thorough understanding of machine learning techniques and model evaluation metrics. The process begins with identifying the right algorithms that suit the specific business problem. Algorithms like decision trees, neural networks, and support vector machines have different strengths and applications, and choosing the right one is crucial for effective model performance.
Once the algorithm is selected, the next step is training the model using relevant datasets. This involves feeding the algorithm with historical data to learn patterns and make predictions. Data preprocessing is a critical phase here, where data is cleaned, normalized, and sometimes augmented to ensure the model receives high-quality inputs.
Model training is an iterative process. Initial models are often imperfect and require continuous refinement. Hyperparameter tuning, a process of adjusting the parameters that control the learning process, is used to improve model performance. Techniques like grid search and random search can be employed to find the optimal set of hyperparameters.
Businesses should adopt an iterative approach to model development, continuously refining and improving the models based on feedback and performance metrics. This helps in ensuring that the models are accurate, reliable, and aligned with business objectives. Regular updates and retraining are also necessary to keep the model relevant as new data becomes available.
Example: A retail company developing a recommendation system would start by selecting a collaborative filtering algorithm. The model would be trained using past purchase data to predict future customer preferences. The initial recommendations might be refined by continuously feeding the model new purchase data and adjusting the algorithm's parameters.
Testing and Validating AI Solutions
Testing and validating AI solutions are critical for ensuring their effectiveness and reliability. This involves conducting rigorous testing on different datasets, scenarios, and conditions to identify any potential issues or biases.
Validation techniques such as cross-validation, A/B testing, and performance benchmarking can help in assessing the accuracy and robustness of AI models. Cross-validation, for instance, involves splitting the dataset into multiple parts and training the model on each part while validating on the remaining parts. This helps in understanding how the model generalizes to unseen data.
A/B testing, commonly used in marketing and web development, can be employed to compare the performance of the AI model against a baseline or another model. This method helps in quantifying the model's impact on business metrics. Performance benchmarking involves comparing the model's performance against industry standards or similar models to gauge its effectiveness.
Businesses should also involve end-users in the testing process to gather feedback and ensure that the solutions meet their needs. This can be done through pilot programs where the AI solution is deployed in a controlled environment, and user interactions are monitored and analyzed.
Example: A financial institution implementing an AI-based fraud detection system would use historical transaction data for cross-validation. They might also run A/B tests to compare the AI system's performance against the existing manual fraud detection process. User feedback from fraud analysts would be crucial in refining the system.
Scaling Successful AI Projects Across the Organization
Once AI projects demonstrate success in pilot phases, businesses can scale them across the organization. This involves deploying the AI solutions to different departments, locations, and use cases, ensuring that they are integrated seamlessly into existing workflows.
Scaling AI projects requires careful planning, coordination, and resource allocation. Businesses should establish clear guidelines and best practices for scaling AI solutions, ensuring that they are adopted consistently across the organization. This includes developing comprehensive documentation, training programs for employees, and robust support systems to address any issues that arise during the scaling process.
Steps to Scale AI Projects:
Assessment of Scalability: Evaluate the technical and operational scalability of the AI solution. Ensure that the infrastructure can handle increased loads and that the solution integrates well with existing systems.
Standardization: Develop standardized processes for model deployment, monitoring, and maintenance. This ensures consistency and reliability across different implementations.
Training and Support: Provide comprehensive training for employees to ensure they understand how to use and interact with the AI solution. Establish support teams to assist with any technical issues.
Monitoring and Optimization: Continuously monitor the performance of the AI solution as it is scaled across the organization. Gather feedback and make necessary adjustments to optimize its performance.
Example: An e-commerce company that successfully piloted an AI-based inventory management system in one warehouse might scale the system to all its warehouses. This would involve ensuring that the system integrates with different inventory management software, training warehouse staff, and establishing a support team to handle technical issues.
Measuring AI Impact
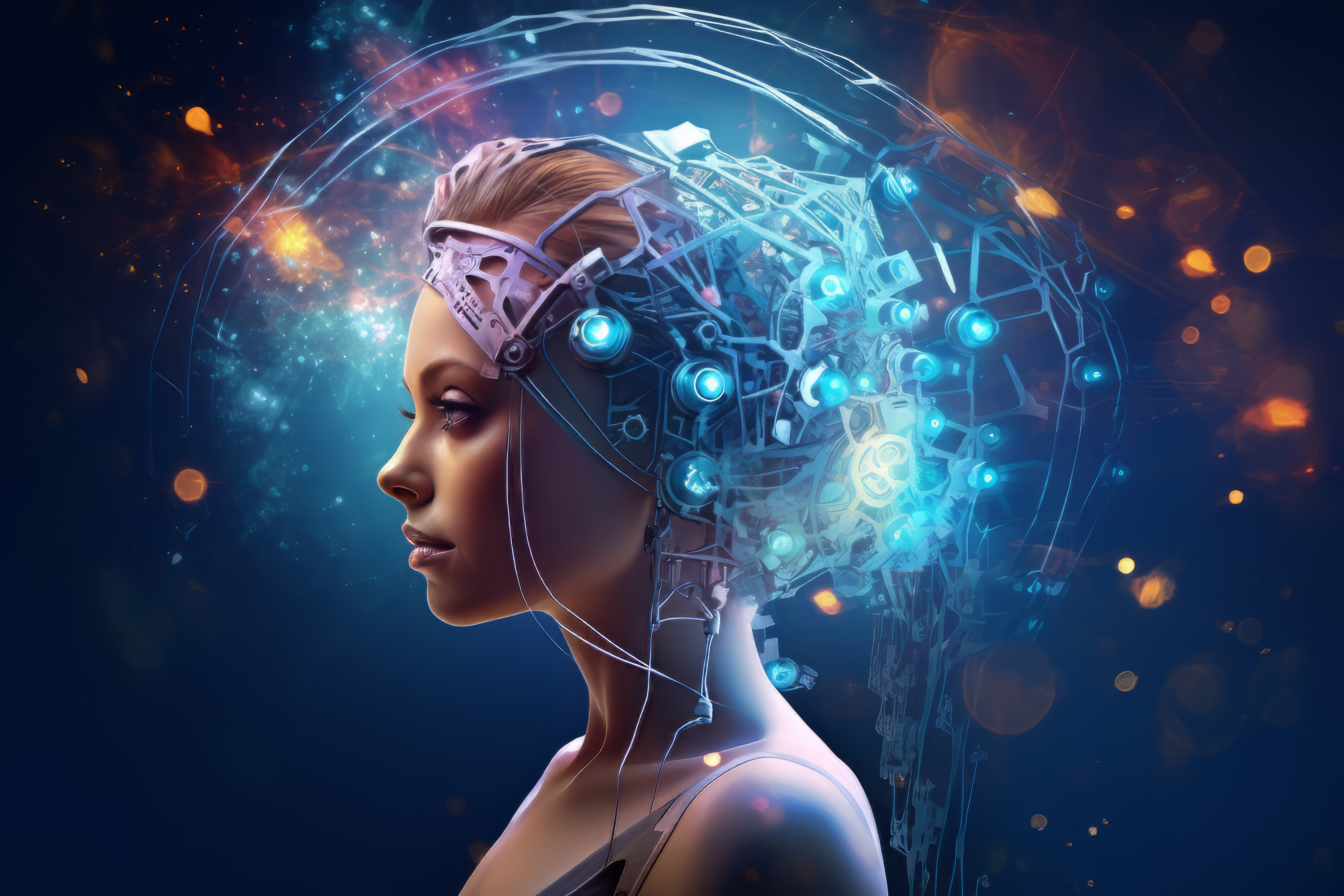
Defining Key Performance Indicators (KPIs) for AI Projects
Defining KPIs for AI projects is essential for measuring their impact and success. KPIs should be aligned with business goals and provide a clear indication of the value generated by AI initiatives . Common KPIs for AI projects include accuracy, precision, recall, ROI, and user satisfaction.
Steps to Define KPIs:
Align with Business Goals: Ensure that KPIs are directly linked to the business objectives the AI project aims to achieve. For example, an AI-powered customer service chatbot's KPIs might include response time, resolution rate, and customer satisfaction.
Quantifiable Metrics: Choose KPIs that are quantifiable and can be measured accurately. This allows for objective evaluation of the AI project's performance.
Regular Monitoring: Establish a framework for tracking and reporting KPIs regularly. This helps in identifying trends, assessing performance, and making data-driven decisions.
Example: A healthcare provider implementing an AI system for diagnosing medical conditions might define KPIs such as diagnostic accuracy, time to diagnosis, and patient outcomes. These KPIs would help evaluate the system's effectiveness in improving patient care.
Monitoring AI Performance and Outcomes
Monitoring AI performance involves continuously tracking the behavior and outcomes of AI models. This includes monitoring their accuracy, efficiency, and alignment with business objectives. Businesses should implement monitoring tools and dashboards to provide real-time insights into AI performance.
Regular performance reviews and audits can help in identifying any issues or deviations from expected outcomes. This allows businesses to take corrective actions and ensure that AI models continue to deliver value.
Steps to Monitor AI Performance:
Real-Time Monitoring: Use monitoring tools to track AI model performance in real-time. This helps in detecting issues early and making timely adjustments.
Performance Reviews: Conduct regular reviews to evaluate the AI model's performance against predefined KPIs. Analyze trends and identify areas for improvement.
User Feedback: Gather feedback from end-users to understand their experience with the AI solution. Use this feedback to make necessary adjustments and improvements.
Example: An AI-driven marketing automation platform might use real-time monitoring to track campaign performance, such as click-through rates and conversion rates. Regular performance reviews and user feedback would help optimize the platform for better results.
Making Data-Driven Decisions Based on AI Insights
One of the key benefits of AI is its ability to provide data-driven insights that inform decision-making. Businesses should leverage AI-generated insights to make informed decisions, optimize processes, and identify new opportunities.
This involves integrating AI insights into business intelligence systems, decision-support tools, and strategic planning processes. By making data-driven decisions, businesses can enhance their agility, responsiveness, and competitiveness.
Steps to Make Data-Driven Decisions:
Integrate AI Insights: Ensure that AI-generated insights are integrated into business intelligence systems and decision-support tools. This provides a comprehensive view of the data and facilitates informed decision-making.
Analyze Trends: Use AI insights to identify trends and patterns that can inform strategic decisions. This helps in understanding market dynamics, customer behavior, and operational efficiency.
Optimize Processes: Leverage AI insights to optimize business processes, improve efficiency, and reduce costs. This can involve automating repetitive tasks, streamlining workflows, and enhancing resource allocation.
Identify Opportunities: Use AI insights to identify new opportunities for growth and innovation. This can include exploring new markets, developing new products, and improving customer engagement.
Example: A retail company might use AI-generated insights to optimize inventory management. By analyzing sales data and predicting demand patterns, the company can make data-driven decisions to reduce stockouts and overstock situations, improving overall efficiency and customer satisfaction.
By following these steps, businesses can effectively implement and scale AI projects, measure their impact, and make data-driven decisions to achieve their strategic goals. This comprehensive approach ensures that AI initiatives are successful and deliver significant value to the organization.
Ethics and Governance
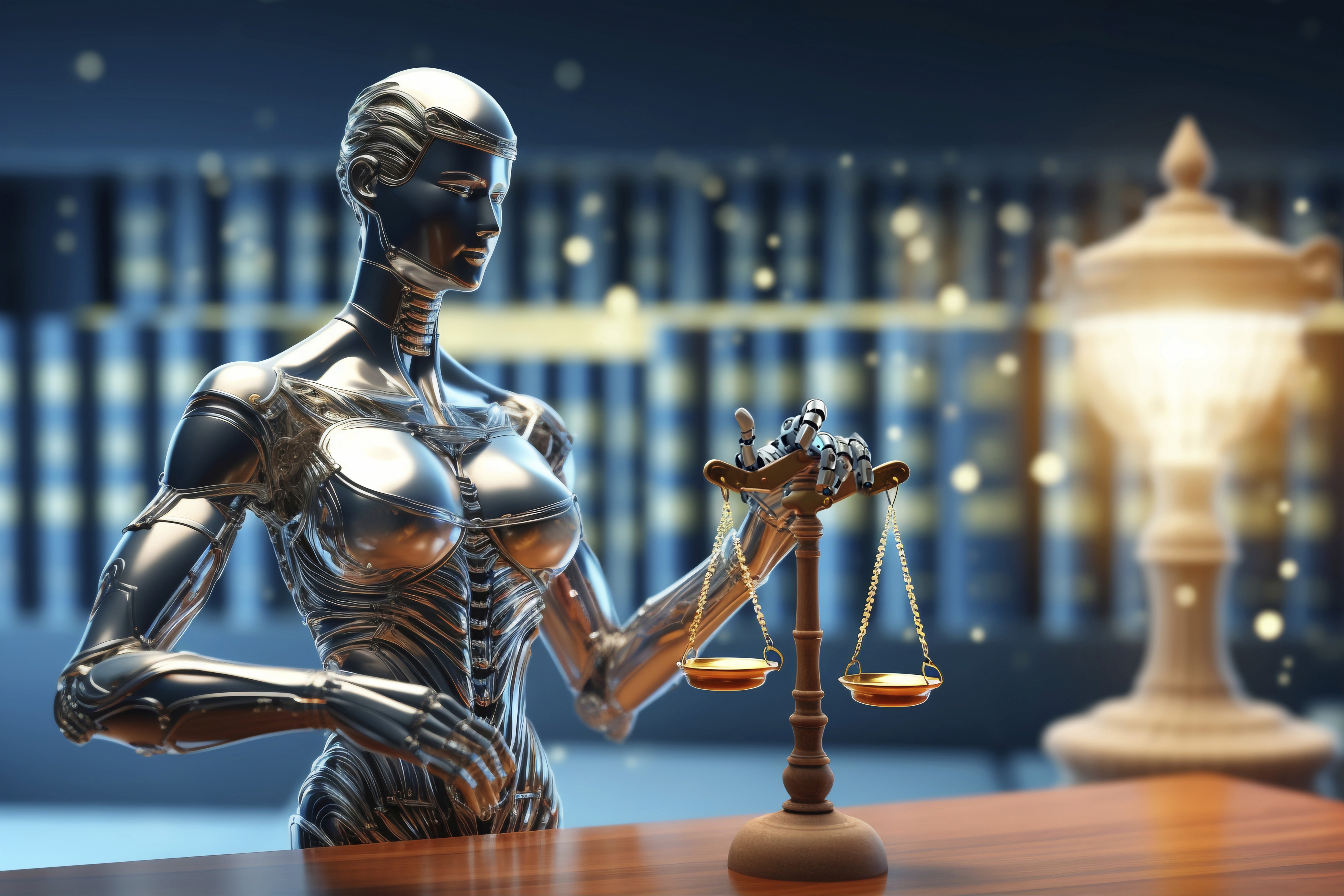
Ensuring Ethical AI Practices
Ethical considerations are paramount in AI implementation. Businesses must ensure that their AI practices are ethical, transparent, and fair. This involves addressing issues such as bias, discrimination, and privacy concerns.
To achieve this, companies should adopt ethical AI principles such as fairness, accountability, transparency, and privacy. Regular audits and assessments of AI systems can help identify and mitigate biases. Engaging stakeholders, including employees, customers, and regulators, in discussions on ethical AI practices is crucial to build trust and ensure compliance with ethical standards.
Fairness: Ensuring that AI models do not discriminate based on race, gender, or other protected characteristics.
Accountability: Establishing clear accountability for AI decisions and outcomes.
Transparency: Making AI systems and their decision-making processes transparent to users and stakeholders.
Privacy: Protecting the privacy of individuals by securing personal data and ensuring compliance with data protection regulations like GDPR and CCPA.
Example: IBM’s principles for trust and transparency in AI emphasize the importance of explainability, fairness, and accountability in AI systems .
Establishing AI Governance Frameworks
Effective AI governance is essential to manage the risks and ensure the responsible use of AI. This involves establishing clear policies, procedures, and oversight mechanisms to govern AI activities. An AI governance framework should include:
Policy Development: Establishing AI policies that define acceptable use, data management practices, and ethical guidelines.
Roles and Responsibilities: Defining roles and responsibilities for AI governance, including the appointment of AI ethics officers and governance committees.
Risk Management: Implementing risk management processes to identify, assess, and mitigate risks associated with AI deployment.
Monitoring and Compliance: Setting up mechanisms to monitor AI performance and ensure compliance with policies and regulations.
Example: Google’s AI principles guide the ethical development and use of AI, emphasizing responsible AI governance and adherence to ethical standards .
Managing AI Risks and Compliance
AI systems can pose various risks, including operational, reputational, and legal risks. Businesses must proactively manage these risks to prevent negative outcomes. This involves:
Risk Assessment: Conducting thorough risk assessments during the development and deployment of AI systems.
Mitigation Strategies: Implementing mitigation strategies to address identified risks, such as bias mitigation techniques and robust security measures.
Compliance: Ensuring compliance with relevant regulations and standards, such as data protection laws (e.g., GDPR, CCPA) and industry-specific regulations.
Regular training and awareness programs can help employees understand the risks associated with AI and the importance of compliance with ethical and legal standards.
Example: Microsoft's AI governance framework includes a comprehensive risk management process to address potential risks associated with AI deployment .
Success Stories
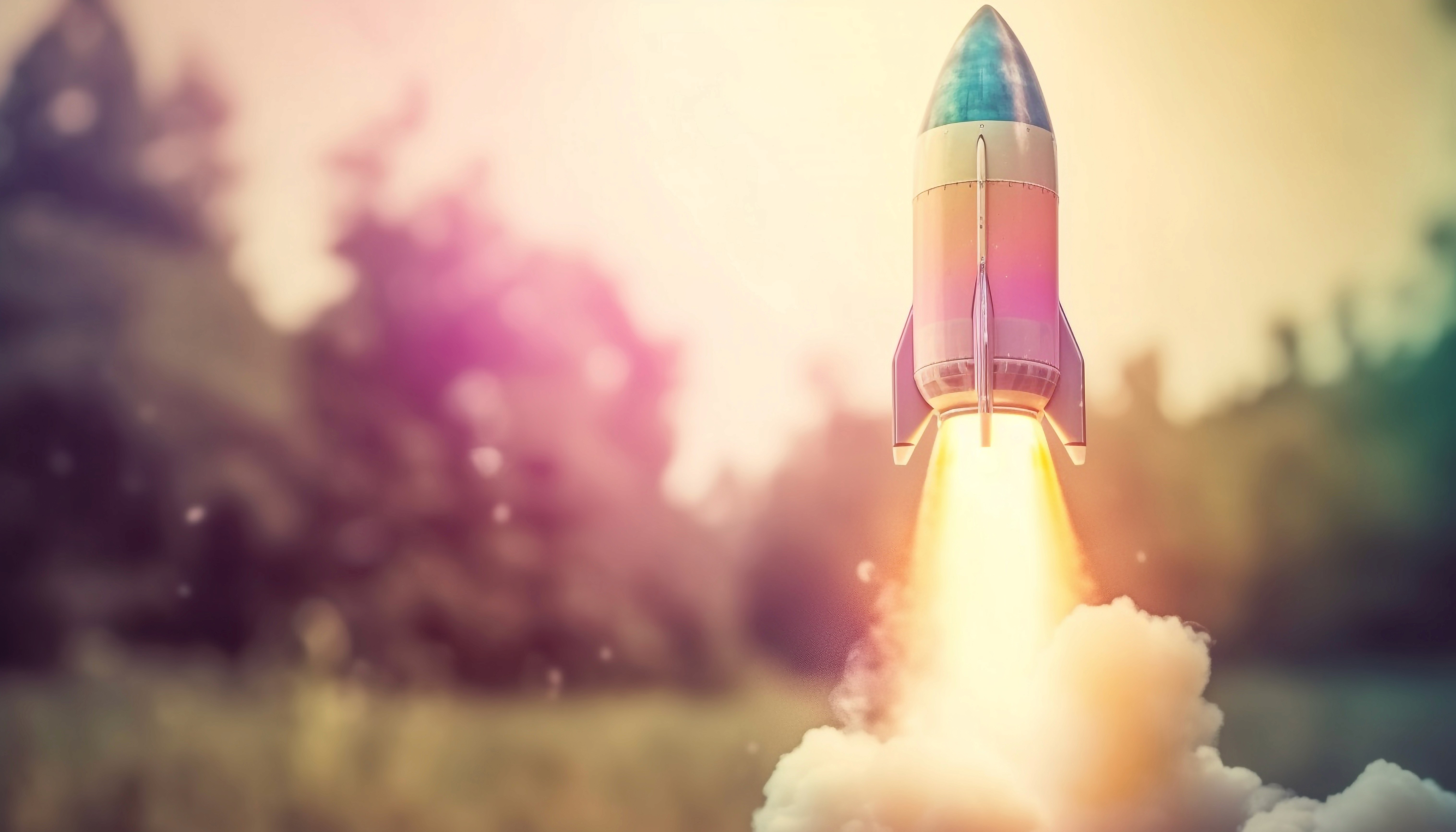
Case Study 1: AI in Retail
A leading U.S. retail company implemented an AI-driven customer personalization strategy to enhance customer experience and boost sales. The AI system analyzed customer data, including purchase history and browsing behavior, to provide personalized product recommendations. This resulted in a 20% increase in sales and a 15% improvement in customer satisfaction.
Key Takeaways:
Personalization can significantly enhance customer experience and drive sales.
Data-driven insights enable businesses to understand customer preferences and tailor offerings accordingly.
Case Study 2: AI in Manufacturing
A U.S.-based manufacturing firm adopted predictive maintenance AI solutions to reduce downtime and maintenance costs. The AI system monitored equipment performance in real-time, predicting failures before they occurred and scheduling maintenance proactively. This led to a 25% reduction in maintenance costs and a 30% decrease in unplanned downtime.
Key Takeaways:
Predictive maintenance can lead to significant cost savings and improved operational efficiency.
Real-time monitoring and predictive analytics enable proactive maintenance, reducing downtime and enhancing productivity.
Case Study 3: AI in Financial Services
A financial services company implemented an AI-powered fraud detection system to enhance security and protect customer assets. The AI system analyzed transaction patterns and identified suspicious activities in real-time, preventing fraudulent transactions. This resulted in a 40% reduction in fraud-related losses and a 50% improvement in detection accuracy.
Key Takeaways:
AI can significantly enhance security and fraud detection capabilities.
Real-time analysis and anomaly detection enable timely identification and prevention of fraudulent activities.
Conclusion
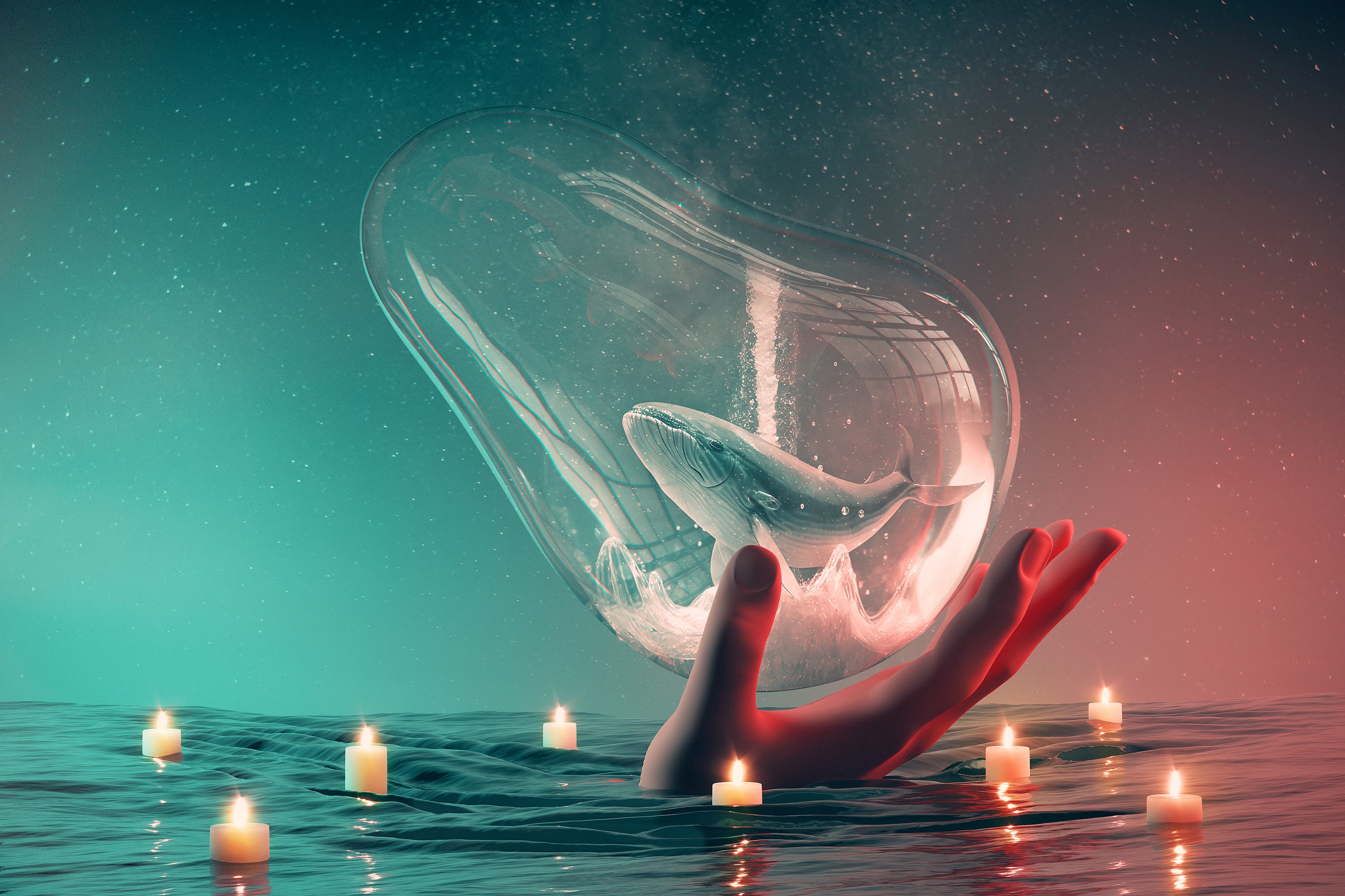
Implementing an AI strategy can transform U.S. businesses, driving innovation, efficiency, and growth. By following a comprehensive approach that includes strategic planning, robust infrastructure, skilled talent, ethical governance, and continuous learning, businesses can successfully navigate the complexities of AI adoption and maximize its benefits.
As we look to the future, AI will continue to play a pivotal role in shaping the business landscape. By embracing AI, businesses can unlock new opportunities, achieve their strategic goals, and stay competitive in an increasingly digital world.
The Long-Term Benefits of an AI Strategy
Enhanced Productivity: Automating routine tasks frees up employees to focus on higher-value activities.
Improved Decision-Making: AI provides data-driven insights that inform strategic decisions.
Cost Savings: AI can optimize operations, reduce waste, and lower operational costs.
Competitive Advantage: Businesses that leverage AI can stay ahead of competitors by innovating faster and more effectively.
Preparing for the Future of AI in Business
Investing in R&D: Allocating resources for AI research and development to explore new technologies and applications.
Collaborating with AI Experts: Partnering with AI research institutions, startups, and experts to access cutting-edge knowledge and technologies.
Continuous Learning: Encouraging a culture of continuous learning and innovation to keep up with the latest developments in AI.
Ensuring Sustainable and Ethical AI Growth: Promoting ethical AI practices, fostering inclusive AI, and adopting sustainable practices in AI development and deployment.
By focusing on these areas, businesses can build a solid foundation for AI adoption, ensuring that they are well-positioned to take advantage of the opportunities that AI offers while mitigating potential risks. The future of AI in business is bright, and those who invest in and embrace this technology today will be the leaders of tomorrow.
Recent Articles
Feb 21, 2025
Household Management Made Easy
Proven Strategies for a More Organized and Stress-Free Home
Feb 7, 2015
Boost Your Focus and Productivity with AI
The Role of AI and Neurotechnology in Productivity Enhancement
Jan 24, 2025
The Science of Focus for Productivity:
Brain Hacks to Stay Productive in the Age of Distraction
Jan 13, 2025